Dragon king theory
Dragon king (DK) is a double metaphor for an event that is both extremely large in size or impact (a "king") and born of unique origins (a "dragon") relative to its peers (other events from the same system). DK events are generated by or correspond to mechanisms such as positive feedback, tipping points, bifurcations, and phase transitions, that tend to occur in nonlinear and complex systems, and serve to amplify DK events to extreme levels. By understanding and monitoring these dynamics, some predictability of such events may be obtained.[1][2][3]
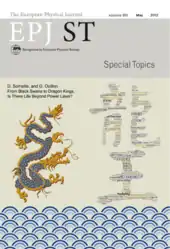
The dragon king theory was developed by Didier Sornette, who hypothesizes that many crises are in fact DKs rather than black swans—i.e., they may be predictable to some degree. Given the importance of crises to the long-term organization of a variety of systems, the DK theory urges that special attention be given to the study and monitoring of extremes, and that a dynamic view be taken. From a scientific viewpoint, such extremes are interesting because they may reveal underlying, often hidden, organizing principles. Practically speaking, one should study extreme risks, but not forget that significant uncertainty will almost always be present, and should be rigorously considered in decisions regarding risk management and design.
The DK theory is related to concepts such as black swan theory, outliers, complex systems, nonlinear dynamics, power laws, extreme value theory, prediction, extreme risks, and risk management.
Black swans and dragon kings
A black swan can be considered a metaphor for an event that is surprising (to the observer), has a major effect, and, after being observed, is rationalized in hindsight. The theory of black swans is epistemological, relating to the limited knowledge and understanding of the observer. The term was introduced and popularized by Nassim Taleb and has been associated with concepts such as heavy tails, non-linear payoffs, model error, and even Knightian uncertainty, whose "unknowable unknown" event terminology was popularized by former United States Secretary of Defense Donald Rumsfeld. Taleb claims that black swan events are not predictable, and in practice, the theory encourages one to "prepare rather than predict", and limit one's exposure to extreme fluctuations.
The black swan concept is important and poses a valid criticism of people, firms, and societies that are irresponsible in the sense that they are overly confident in their ability to anticipate and manage risk. However, claiming that extreme events are—in general—unpredictable may also lead to a lack of accountability in risk management roles. In fact, it is known that in a wide range of physical systems that extreme events are predictable to some degree.[4][5][2][3] One simply needs to have a sufficiently deep understanding of the structure and dynamics of the focal system, and the ability to monitor it. This is the domain of the dragon kings. Such events have been referred to as "grey swans" by Taleb. A more rigorous distinction between black swans, grey swans, and dragon kings is difficult as black swans are not precisely defined in physical and mathematical terms. However, technical elaboration of concepts in the Black Swan book are elaborated in the Silent Risk document. An analysis of the precise definition of a black swan in a risk management context was written by professor Terje Aven.[6]
Beyond power laws
It is well known that many phenomena in both the natural and social sciences have power law statistics (Pareto distribution).[8][9][10] Furthermore, from extreme value theory, it is known that a broad range of distributions (the Frechet class) have tails that are asymptotically power law. The result of this is that, when dealing with crises and extremes, power law tails are the "normal" case. The unique property of power laws is that they are scale-invariant, self-similar and fractal. This property implies that all events—both large and small—are generated by the same mechanism, and thus there will be no distinct precursors by which the largest events may be predicted. A well-known conceptual framework for events of this type is self-organized criticality. Such concepts are compatible with the theory of the black swan. However Taleb has also stated that considering the power law as a model instead of a model with lighter tails (e.g., a Gaussian) "converts black swans into gray ones", in the sense that the power law model gives non-negligible probability to large events.
In a variety of studies it has been found that, despite the fact that a power law models the tail of the empirical distribution well, the largest events are significantly outlying (i.e., much larger than what would be expected under the model).[7][11][12] Such events are interpreted as dragon kings as they indicate a departure from the generic process underlying the power law. Examples of this include the largest radiation release events occurring in nuclear power plant accidents, the largest city (agglomeration) within the sample of cities in a country, the largest crashes in financial markets, and intraday wholesale electricity prices.[7][13]
Mechanisms
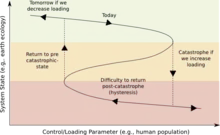
Physically speaking, dragon kings may be associated with the regime changes, bifurcations, and tipping points of complex out-of-equilibrium systems.[1] For instance, the catastrophe (fold bifurcation) of the global ecology illustrated in the figure could be considered to be a dragon king: Many observers would be surprised by such a dramatic change of state. However, it is well known that in dynamic systems, there are many precursors as the system approaches the catastrophe.
Positive feedback is also a mechanism that can spawn dragon kings. For instance, in a stampede the number of cattle running increases the level of panic which causes more cattle to run, and so on. In human dynamics such herding and mob behavior has also been observed in crowds, stock markets, and so on (see herd behavior).
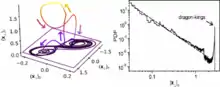
Dragon kings are also caused by attractor bubbling in coupled oscillator systems.[15] Attractor bubbling is a generic behavior appearing in networks of coupled oscillators where the system typically orbits in an invariant manifold with a chaotic attractor (where the peak trajectories are low), but is intermittently pushed (by noise) into a region where orbits are locally repelled from the invariant manifold (where the peak trajectories are large). These excursions form the dragon kings, as illustrated in the figure. It is claimed that such models can describe many real phenomena such as earthquakes, brain activity, etc.[15] A block and spring mechanical model, considered as a model of geological faults and their earthquake dynamics, produced a similar distribution.[16]
It could also be the case that dragon kings are created as a result of system control or intervention. That is, trying to suppress the release of stress or death in dynamic complex systems may lead to an accumulation of stress or a maturation towards instability. For instance, brush/forest fires are a natural occurrence in many areas. Such fires are inconvenient and thus we may wish that they are diligently extinguished. This leads to long periods without inconvenient fires, however, in the absence of fires, dead wood accumulates. Once this accumulation reaches a critical point, and a fire starts, the fire becomes so large that it cannot be controlled—a singular event that could be considered to be a dragon king. Other policies, such as doing nothing (allowing for small fires to occur naturally), or performing strategic controlled burning, would avoid enormous fires by allowing for frequent small ones. Another example is monetary policy. Quantitative easing programs and low interest rate policies are common, with the intention of avoiding recessions, promoting growth, etc. However, such programs build instability by increasing income inequality, keeping weak firms alive, and inflating asset bubbles.[17][18] Ultimately such policies, aimed at smoothing out economic fluctuations, will enable an enormous correction—a dragon king.
Detecting DKs as statistical outliers
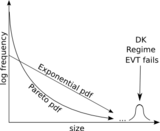
DKs are outliers by definition. However, when calling DKs outliers there is an important proviso: In standard statistics outliers are typically erroneous values and are discarded, or statistical methods are chosen that are somehow insensitive to outliers. Contrarily, DKs are outliers that are highly informative, and should be the focus of much statistical attention. Thus a first step is identifying DKs in historical data. Existing tests are either based on the asymptotic properties of the empirical distribution function (EDF)[13] or on an assumption about the underlying cumulative distribution function (CDF) of the data.[7]
It turns out that testing for outliers relative to an exponential distribution is very general. The latter follows from the Pickands–Balkema–de Haan theorem of extreme value theory which states that a wide range of distributions asymptotically (above high thresholds) have exponential or power law tails. As an aside, this is one explanation why power law tails are so common when studying extremes. To finish the point, since the natural logarithm of a power law tail is exponential, one can take the logarithm of power law data and then test for outliers relative to an exponential tail. There are many test statistics and techniques for testing for outliers in an exponential sample. An inward test sequentially tests the largest point, then the second largest, and so on, until the first test that is not rejected (i.e., the null hypothesis that the point is not an outlier is not rejected). The number of rejected tests identifies the number of outliers. For instance, where is the sorted sample, the inward robust test uses the test statistic where r is the point being tested , and where m is the pre-specified maximum number of outliers. At each step the p-value for the test statistic must be computed and, if lower than some level, the test rejected. This test has many desirable properties: It does not require that the number of outliers be specified, it is not prone to under (masking) and over (swamping) estimation of the number outliers, it is easy to implement, and the test is independent of the value of the parameter of the exponential tail.[7]
Examples
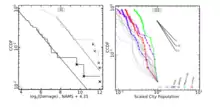
Some examples of where dragon kings have been detected as outliers include:[7][13]
- financial crashes as measured by drawdowns, where the outliers correspond to terrorist attacks (e.g., the 2005 London bombing), and the flash crash of 2010;
- the radiation released and financial losses caused by accidents at nuclear power plants, where outliers correspond to runaway disasters where safety mechanisms were overwhelmed;
- the largest city (measured by the population in its agglomeration) in the population of cities within a country, where the largest city plays a disproportionately important role in the dynamics of the country, and benefits from unique growth;
- intraday wholesale electricity prices; and
- three-wave nonlinear interaction—it is possible to suppress the emergence of dragon kings.[19]
Modeling and prediction
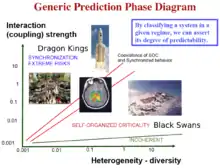
How one models and predicts dragon kings depends on the underlying mechanism. However, the common approach will require continuous monitoring of the focal system and comparing measurements with a (non-linear or complex) dynamic model. It has been proposed that the more homogeneous the system, and the stronger its interactions, the more predictable it will be.[20]
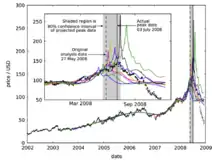
For instance, in non-linear systems with phase transitions at a critical point, it is well known that a window of predictability occurs in the neighborhood of the critical point due to precursory signs: the system recovers more slowly from perturbations, autocorrelation changes, variance increases, spatial coherence increases, etc.[22][23] These properties have been used for prediction in many applications ranging from changes in the bio-sphere[14] to rupture of pressure tanks on the Ariane rocket.[24]
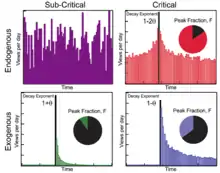
For the phenomena of unsustainable growth (e.g., of populations or stock prices), one can consider a growth model that features a finite time singularity, which is a critical point where the growth regime changes. In systems that are discrete scale invariant such a model is power law growth, decorated with a log-periodic function.[26][27] Fitting this model on the growth data (non-linear regression) allows for the prediction of the singularity, i.e., the end of unsustainable growth. This has been applied to many problems,[3] for instance: rupture in materials,[24][28] earthquakes,[29] and the growth and burst of bubbles in financial markets[12][30][31][32][33]
An interesting dynamic to consider, that may reveal the development of a block-buster success, is epidemic phenomena: e.g., the spread of plague, viral phenomena in media, the spread of panic and volatility in stock markets, etc. In such a case, a powerful approach is to decompose activity/fluctuations into exogeneous and endogeneous parts, and learn about the endogenous dynamics that may lead to high impact bursts in activity.[25][34][35]
Prediction and decision-making
Given a model and data, one can obtain a statistical model estimate. This model estimate can then be used to compute interesting quantities such as the conditional probability of the occurrence of a dragon king event in a future time interval, and the most probable occurrence time. When doing statistical modeling of extremes, and using complex or nonlinear dynamic models, there is bound to be substantial uncertainty. Thus, one should be diligent in uncertainty quantification: not only considering the randomness present in the fitted stochastic model, but also the uncertainty of its estimated parameters (e.g., with Bayesian techniques or by first simulating parameters and then simulating from the model with those parameters), and the uncertainty in model selection (e.g., by considering an ensemble of different models).
One can then use the estimated probabilities and their associated uncertainties to inform decisions. In the simplest case, one performs a binary classification: predicting that a dragon king will occur in a future interval if its probability of occurrence is high enough, with sufficient certainty. For instance, one may take a specific action if a dragon king is predicted to occur. An optimal decision will then balance the cost of false negatives/false positives and misses/false alarms according to a specified loss function. For instance, if the cost of a miss is very large relative to the cost of a false alarm, the optimal decision will detect dragon kings more frequently than they occur. One should also study the true positive rate of the prediction. The smaller this value is, the weaker the test, and the closer one is to black swan territory. In practice the selection of the optimal decision, and the computation of its properties must be done by cross validation with historical data (if available), or on simulated data (if one knows how to simulate the dragon kings).
In a dynamic setting the dataset will grow over time, and the model estimate, and its estimated probabilities will evolve. One may then consider combining the sequence of estimates/probabilities when performing prediction. In this dynamic setting, the test will likely be weak most of the time (e.g., when the system is around equilibrium), but as one approaches a dragon king, and precursors become visible, the true positive rate should increase.
The importance of extreme risks
Dragon kings form special kinds of events leading to extreme risks (which can also be opportunities). That extreme risks are important should be self-evident. Natural disasters provide many examples (e.g., asteroid impacts leading to extinction). Some statistical examples of the impact of extremes are that: the largest nuclear power plant accident (2011 Fukushima disaster) caused more damage than all (>200) other historical accidents together,[36] the largest 10 percent of private data breaches from organizations accounts for 99 percent of the total breached private information,[37] the largest five epidemics since 1900 caused 20 times the fatalities of the remaining 1363,[7][38] etc. In general such statistics arrive in the presence of heavy-tailed distributions, and the presence of dragon kings will augment the already oversized impact of extreme events.
Despite the importance of extreme events, due to ignorance, misaligned incentives, and cognitive biases, there is often a failure to adequately anticipate them. Technically speaking, this leads to poorly specified models where distributions that are not heavy-tailed enough, and under-appreciate both serial and multivariate dependence of extreme events. Some examples of such failures in risk assessment include the use of Gaussian models in finance (Black–Scholes, the Gaussian copula, LTCM), the use of Gaussian processes and linear wave theory failing to predict the occurrence of rogue waves, the failure of economic models in general to predict the financial crisis of 2007–2008, and the under-appreciation of external events, cascades, and nonlinear effects in probabilistic risk assessment, leading to not anticipating the Fukushima Daiichi nuclear disaster in 2011. Such high impact failures emphasize the importance of the study of extremes.
Risk management
The dragon king concept raises many questions about how one can deal with risk. Of course, if possible, exposure to large risks should be avoided (often referred to as the "black swan approach"). However, in many developments, exposure to risk is a necessity, and a trade-off between risk and return needs to be navigated.
In an adaptive system, where prediction of dragon kings is successful, one can act to defend the system or even profit. How to design such resilient systems, as well as their real time risk monitoring systems,[39] is an important and interdisciplinary problem where dragon kings must be considered.
On another note, when it comes to the quantification of risk in a given system (whether it be a bank, an insurance company, a dike, a bridge, or a socio-economic system), risk needs to be accounted for over a period, such as annually. Typically one is interested in statistics such as the annual probability of loss or damage in excess of some value (value at risk), other tail risk measures, and return periods. To provide such risk characterizations, the dynamic dragon kings must be reasoned about in terms of annual frequency and severity statistics. These frequency and severity statistics can then be brought together in a model such as a compound Poisson process.
Provided that the statistical properties of the system are consistent over time (stationary), frequency and severity statistics may be constructed based on past observations, simulations, and/or assumptions. If not, one may only construct scenarios. However, in any case, given the uncertainty present, a range of scenarios should be considered. Due to the shortage of data for extreme events, the principle of parsimony, and theoretical results from extreme value theory about universal tail models, one typically relies on a generalized Pareto distribution (GPD) tail model. However such a model excludes DKs. Thus, when one has sufficient reason to believe that DKs are present, or if one simply wants to consider a scenario, one may e.g., consider a density mixture of a GPD and a density for the DK regime.
References
- Sornette, Didier, and Guy Ouillon. "Dragon-kings: mechanisms, statistical methods and empirical evidence." The European Physical Journal Special Topics 205.1 (2012): 1–26.
- D. Sornette, Dragon-Kings, Black Swans and the Prediction of Crises, International Journal of Terraspace Science and Engineering 1(3), 1–17 (2009) (https://arxiv.org/abs/0907.4290) and (http://ssrn.com/abstract=1470006)
- D. Sornette, Predictability of catastrophic events: material rupture, earthquakes, turbulence, financial crashes and human birth, Proc. Nat. Acad. Sci. USA 99, SUPP1 (2002), 2522–2529.
- Didier Sornette TED Talk: https://www.ted.com/talks/didier_sornette_how_we_can_predict_the_next_financial_crisis?language=en
- Albeverio, Sergio, Volker Jentsch, and Holger Kantz. Extreme events in nature and society. Springer Science & Business Media, 2006.
- Aven, Terje. "On the meaning of a black swan in a risk context." Safety science 57 (2013): 44–51.
- Wheatley, Spencer, and Didier Sornette. "Multiple Outlier Detection in Samples with Exponential & Pareto Tails: Redeeming the Inward Approach & Detecting Dragon Kings." arXiv preprint arXiv:1507.08689 (2015).
- Mitzenmacher, Michael. "A brief history of generative models for power law and lognormal distributions." Internet mathematics 1.2 (2004): 226–251.
- Newman, Mark EJ. "Power laws, Pareto distributions and Zipf's law." Contemporary physics 46.5 (2005): 323–351.
- Sornette, Didier. "Critical Phenomena in Natural Sciences: Chaos, Fractals, Selforganization and Disorder: Concepts and Tools (Springer Series in Synergetics)." (2006).
- Pisarenko, V. F., and D. Sornette. "Robust statistical tests of Dragon-Kings beyond power law distributions." The European Physical Journal Special Topics 205.1 (2012): 95–115.
- Johansen, Anders, and Didier Sornette. "Shocks, crashes and bubbles in financial markets." Brussels Economic Review (Cahiers economiques de Bruxelles) 53.2 (2010): 201–253.
- Janczura, J.; Weron, R. (2012). "Black swans or dragon-kings? A simple test for deviations from the power law". The European Physical Journal Special Topics. 205 (1): 79–93. arXiv:1102.3712. Bibcode:2012EPJST.205...79J. doi:10.1140/epjst/e2012-01563-9. ISSN 1951-6355.
- Barnosky, Anthony D., et al. "Approaching a state shift in Earth's biosphere."Nature 486.7401 (2012): 52–58.
- Cavalcante, Hugo LD de S., et al. "Predictability and suppression of extreme events in a chaotic system." Physical review letters 111.19 (2013): 198701.
- Shaw, Bruce E., Jean M. Carlson, and James S. Langer. "Patterns of seismic activity preceding large earthquakes." Journal of Geophysical Research: Solid Earth (1978–2012) 97.B1 (1992): 479–488.
- Sornette, Didier, and Peter Cauwels. "1980–2008: The illusion of the perpetual money machine and what it bodes for the future." Risks 2.2 (2014): 103–131.
- Sornette, Didier, and Peter Cauwels. "Managing risk in a creepy world." Journal of Risk Management in Financial Institutions 8.1 (2015): 83–108.
- Viana, Ricardo L.; Caldas, Iberê L.; Iarosz, Kelly C.; Batista, Antonio M.; Szezech Jr, José D.; Santos, Moises S. (1 May 2019). "Dragon-kings death in nonlinear wave interactions". Physica A: Statistical Mechanics and Its Applications. 534: 122296. arXiv:1905.00528. Bibcode:2019PhyA..53422296S. doi:10.1016/j.physa.2019.122296.
- Sornette, D., P. Miltenberger, and C. Vanneste. "Statistical physics of fault patterns self-organized by repeated earthquakes: synchronization versus self-organized criticality." Recent Progresses in Statistical Mechanics and Quantum Field Theory (World Scientific, Singapore, 1995) (1994): 313–332.
- Sornette, Didier, Ryan Woodard, and Wei-Xing Zhou. "The 2006–2008 oil bubble: Evidence of speculation, and prediction." Physica A: Statistical Mechanics and its Applications 388.8 (2009): 1571–1576.
- Strogatz, Steven H. Nonlinear dynamics and chaos: with applications to physics, biology, chemistry, and engineering. Westview press, 2014
- Scheffer, Marten, et al. "Anticipating critical transitions." science 338.6105 (2012): 344–348.
- J.-C. Anifrani, C. Le Floc'h, D. Sornette and B. Souillard, Universal Log-periodic correction to renormalization group scaling for rupture stress prediction from acoustic emissions, J.Phys.I France 5 (6) (1995): 631–638.
- Crane, Riley, and Didier Sornette. "Robust dynamic classes revealed by measuring the response function of a social system." Proceedings of the National Academy of Sciences 105.41 (2008): 15649–15653.
- Sornette, Didier. "Discrete-scale invariance and complex dimensions." Physics reports 297.5 (1998): 239–270.
- Huang, Y., Ouillon, G., Saleur, H., & Sornette, D. (1997). Spontaneous generation of discrete scale invariance in growth models. Physical Review E, 55(6), 6433.
- A. Johansen and D. Sornette, Critical ruptures, Eur. Phys. J. B 18 (2000): 163–181.
- S.G. Sammis and D. Sornette, Positive Feedback, Memory and the Predictability of Earthquakes, Proceedings of the National Academy of Sciences USA 99 SUPP1 (2002): 2501–2508.
- Sornette, Didier, Anders Johansen, and Jean-Philippe Bouchaud. "Stock market crashes, precursors and replicas." Journal de Physique I 6.1 (1996): 167–175.
- Feigenbaum, James A., and Peter GO Freund. "Discrete scale invariance in stock markets before crashes." International Journal of Modern Physics B 10.27 (1996): 3737–3745.
- Sornette, Didier, et al. "Clarifications to questions and criticisms on the Johansen–Ledoit–Sornette financial bubble model." Physica A: Statistical Mechanics and its Applications 392.19 (2013): 4417–4428.
- See http://www.er.ethz.ch/financial-crisis-observatory.html for bubble indicators based on such techniques.
- Sornette, Didier. "Endogenous versus exogenous origins of crises." Extreme events in nature and society. Springer Berlin Heidelberg, 2006. 95–119. (https://arxiv.org/abs/physics/0412026)
- Filimonov, Vladimir, and Didier Sornette. "Quantifying reflexivity in financial markets: Toward a prediction of flash crashes." Physical Review E 85.5 (2012): 056108.
- Wheatley, Spencer, Benjamin Sovacool, and Didier Sornette. "Of Disasters and Dragon Kings: A Statistical Analysis of Nuclear Power Incidents & Accidents." arXiv preprint arXiv:1504.02380 (2015).
- Wheatley, Spencer, Thomas Maillart, and Didier Sornette. "The Extreme Risk of Personal Data Breaches & The Erosion of Privacy." arXiv preprint arXiv:1505.07684 (2015).
- Guha-Sapir, D., R. Below, and Ph Hoyois. "EM-DAT: International disaster database." Univ. Cathol. Louvain, Brussels: Belgium. www. em-dat. net. (2014).
- Sornette, Didier, and Tatyana Kovalenko. "Dynamical Diagnosis and Solutions for Resilient Natural and Social Systems." Planet@ Risk 1 (1) (2013) 7–33.